Remote sensing of Planet Earth – Part 3: Modis vs Sentinel-2
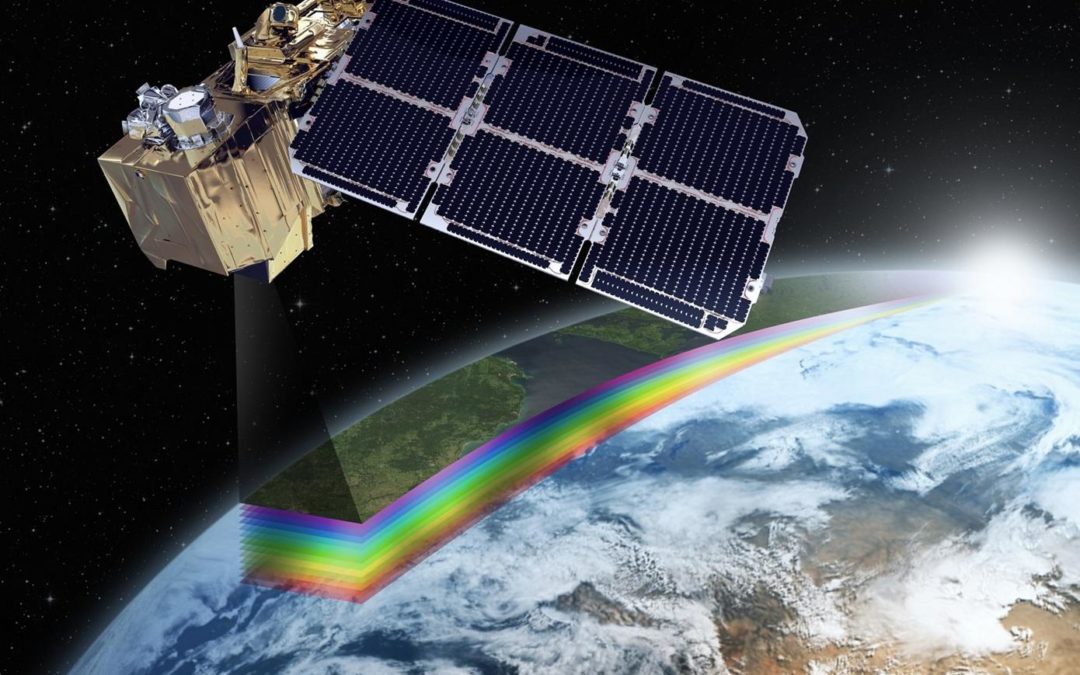
In the last blog post we looked into Sentinel-2 from the European Space Agency. In this one we introduce another optical imagery sensor, called Moderate Resolution Imaging Spectroradiometer (MODIS).
MODIS has some distinctively different properties from Sentinel-2: Sentinel-2 offers higher spatial resolution, while MODIS provides higher temporal and spectral resolutions. Trade-offs between satellites are common and in this post we will explore the impacts of these differences. We start with a look at the products of MODIS.
What is MODIS?
MODIS was brought into space by NASA’s Terra and Aqua satellites in 1999 and 2002. The satellites capture images with 36 spectral bands at a temporal resolution of about 1-2 days and a spatial resolution of up to 250m.
Besides the optical image bands, MODIS provides a lot of processed and derived products. Different levels of products include processing from standard georeferencing to extensive aggregations and transformations specific to an application. With its wide variety of spectral bands, the sensors capture information useful to many different applications, such as land and ocean properties, temperatures at different layers and ozone.
We illustrate two types of products. The first one is MCD43A4, which combines the Terra and Aqua satellites to produce 500m resolution daily images. It corrects the reflectance every day as though the satellites were looking strictly down at the ground, called nadir, by incorporating information over different angles of a sliding window of 16 days.
For the second, we combine the MOD13Q1 and MYD13Q1 products, which are the vegetation index products from the Terra and Aqua satellites respectively. These products precompute the normalised difference vegetation index (NDVI) and enhanced vegetation index (EVI) and create a composite that provides for every 16 days the “best measured” value for each pixel at 250m resolution. The “best” pixel values are determined by an algorithm that filters on cloud and residual atmospheric contamination, and off-nadir angle. The Terra and Aqua satellites generate the same product 8 days apart, such that the combination offers a higher temporal resolution.
The Case for Sentinel-2
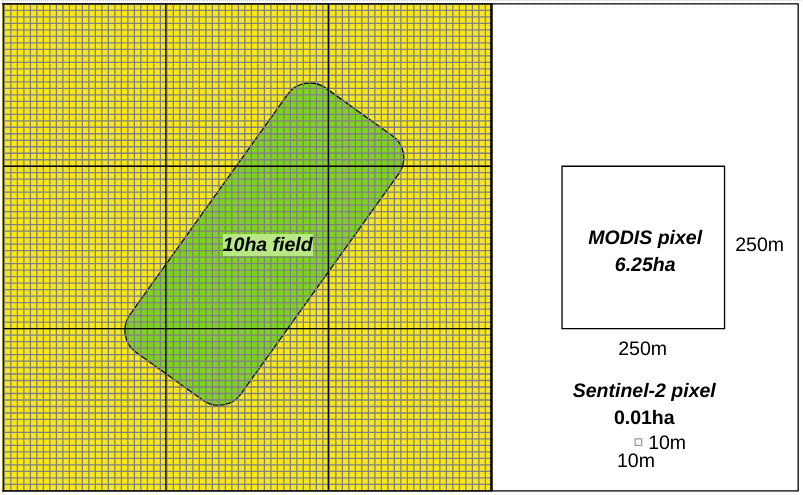
Figure 1: Sketch of a 10ha field overlaid with pixel sizes of MODIS and Sentinel-2
Higher Spatial Resolution
In recent years we have seen great advancements in the spatial resolutions of Earth observation satellites. The spatial resolution of MODIS only goes down to 250m, while the newer Sentinel-2 can reach a 10m resolution. Figures 1 and 2 illustrate the differences in spatial resolution. The green 10 hectares field is overlaid with boxes representing the pixel sizes of a MODIS and Sentinel-2 image. Figure 1 illustrates how most MODIS pixels capture only a small part of the field; notice how all of the pixels capture at least some part outside of the fields.
Choosing pixels to measure the field in such cases is always a trade off. A naive option is to take all pixels that touch the field, which leads to a lot of interference from the NDVI outside of the field in this case. Alternatively, one could decide to take only pixels that are fully contained within a field. However, that can lead to no selected pixels at all. A compromise between these two methods is to take all pixels whose center is contained in the field. In this case that would only include the middle pixel.
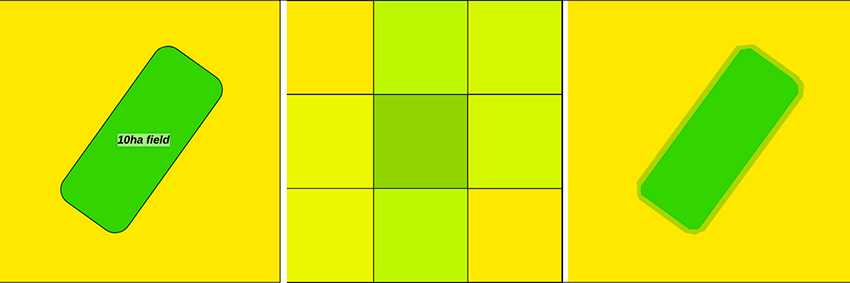
Figure 2: Sketch of figure 1 image measured with 250m and 10m resolution
Left: Actual image, Middle: 250m resolution image, Right: 10m resolution image
Figure 2 shows some sketches of how this NDVI would be altered by the influence of the yellow around the field. Due to its fine pixels, this is much less of an issue for Sentinel-2: Even if some boundary pixels are included, they wouldn’t have a large impact on the overall average of the field.
Thus for any imaging task it is important to be aware of the extents of the targets and the heterogeneity within it, such that one can adapt the spatial resolution necessary. For regional analyses of large forests, small details become less important and one could sacrifice spatial resolution. However, when monitoring differences within individual fields high spatial resolution is key.
Figure 3 displays NDVI time series averaged over a real French field of 5.5 hectares in 2018. Both the daily and the composite MODIS products show a very similar development as the Sentinel-2 series. Still the figure illustrates some natural differences. The MODIS products have to also capture some reflectance outside of the field due to its pixel size. Consequently, the MODIS NDVI is likely to be averaged out a bit more with other ground features, such that the variation is somewhat smaller with less extreme highs and lows.

Figure 3: Average NDVI time series from Sentinel-2 and MODIS over a French wheat field in 2018
The Case for MODIS
Higher Temporal Resolution
While Sentinel-2 takes at least five days to cover the entire globe, MODIS takes only a day or two. These are both relatively high temporal resolutions, which for most applications would be sufficient if the satellites would always capture high quality images. Unfortunately, this is rarely the case. In Northern Europe it is not uncommon that only 30% of measurements are cloud-free. This means that Sentinel-2 would only produce values without clouds once every fifteen days. For certain applications, such as flood mapping, this can be too infrequent.
Higher Spectral Resolution
MODIS measures 36 spectral bands compared to only 13 measured by Sentinel-2. Although they overlap in many regions of the spectrum, a big part of the difference is explained by the higher spectral resolution of MODIS. The spectral resolution describes the ability of a sensor to define fine wavelength intervals. MODIS has very narrow bandwidths, such that it can better distinguish subtle differences in surface reflectance. As illustrated in Figure 4, this allows MODIS to identify the intensity of different shades of blue with different bands for example, while Sentinel-2 captures only an aggregate intensity of these shades all under the same band.
Longer time series
A big pain point of newer high resolution satellites is that they are “too recent” for our data-hungry models. Sentinel-2 was launched in 2015 and MODIS has been running since 1999. Since a satellite can only provide images from its day of launch, MODIS provides a much longer history of data. For events that happen only once a year, like the harvest of a cereal crop, we have only 5 yearly observations from Sentinel-2 to predict the next year. We generally train our models on much more data.
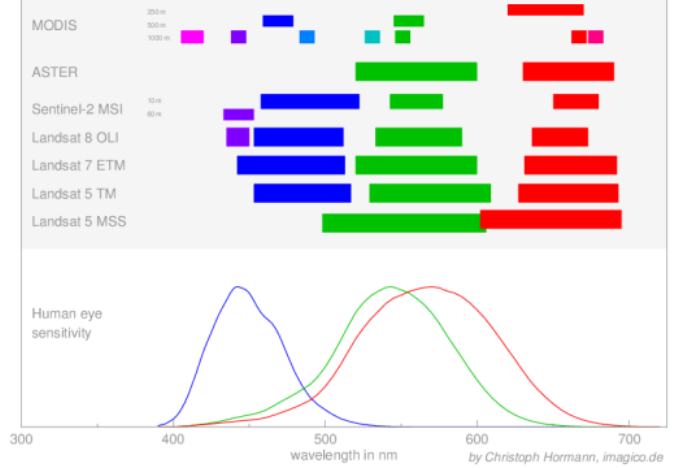
Figure 4: Measured wavelengths by satellite and resolution in the visual range
Source: http://blog.imagico.de/sentinel-3a-satellite-launch/
Conclusion
Neither satellite is definitively better. The right satellite depends on your application. Sentinel-2 provides a much higher spatial resolution to get a better view of the targets. Certain objects, such as smallholder agricultural fields or urban buildings, are too small to be distinguished from MODIS imagery. A very high spatial resolution is then a prerequisite.
If you can sacrifice some of that spatial resolution, MODIS provides very powerful data. A limitation of Sentinel-2 and many other high resolution satellites is that they have been launched quite recently. This restricts the amount of data models can train on, making it harder to identify patterns. MODIS on the other hand provides a much longer time series. It also provides images more frequently, which can be essential for urgent warning systems. With its higher spectral resolution, MODIS can distinguish different colours and other bands better and is more sensitive, which is important in many ocean applications. Lastly, a factor that is commonly neglected is the fact that MODIS provides a lot of the processing. It is time-consuming and costly to retrieve proper information from raw images. The derived products from MODIS allow new users to quickly get started with robust data.
Recent years have seen the launch of many commercial satellites that challenge the spatial resolution even further with resolutions under a meter. Furthermore, there are some attempts to fuse the information of different satellites to exploit the beneficial characteristics of each. At Cervest we work hard to leverage the right information from each satellite for each application.
About us:
We are Owen, Maxim and Ramani, part of the Cervest Science team, a group of statisticians, machine learning & natural scientists, and software engineers. We aim to solve urgent problems within Earth Science through application of machine learning tools and techniques.
If you’re interested in what we do, then please head over to our careers page!
Share this article
Our latest news and insights
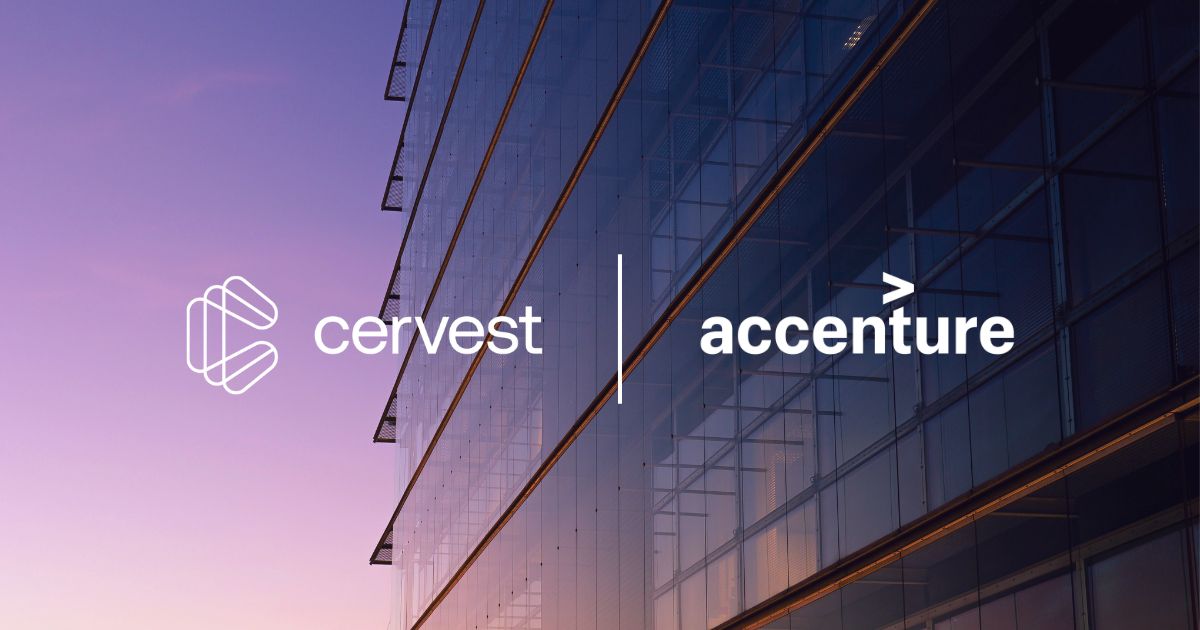
Accenture and Cervest collaborate to bring innovative solutions to clients seeking resilience amid increased climate risk
Read more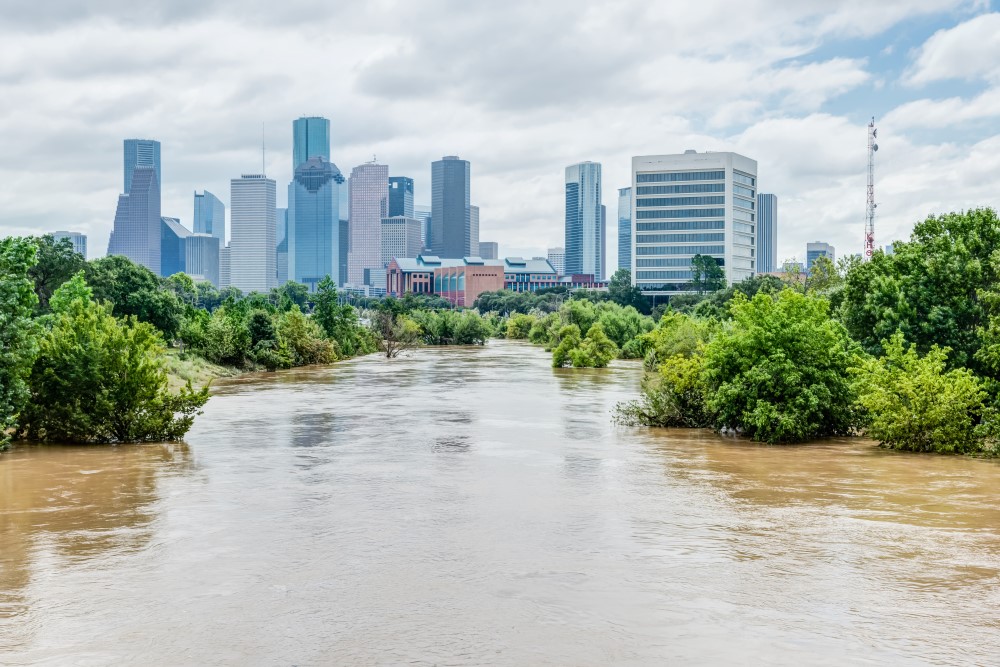
What is climate intelligence and why do businesses and governments need it?
Read more