Cervest @ the Met Office
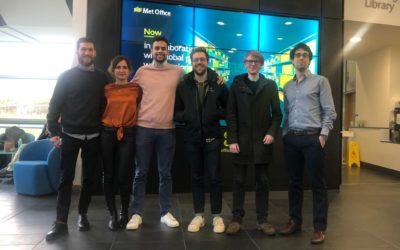

Our team after an intense day at the Met office
From left to right: Federico Roffi, Ernesta Baniulyte, Owen van Eer, Lukas Scholtes, Michael Griffiths and Maxime Rischard.
Weather and climatic data are central to Cervest’s work, feeding into our models and constituting a critical dependency for asset-level risk signals on our open-access beta platform.
A primary provider of such data is the European Centre for Medium-Range Weather Forecasts (ECMWF) and since the UK is a paying member state, the UK Meteorological Office (Met Office) also uses this data in its operations. To better understand the data we use at Cervest, we like to interact with key producers and users of the data. Recently, we had Peter Dueben from the ECMWF visiting to speak about challenges and design choices for global weather and climate models based on machine learning. This time, we sent a modest army of scientists, engineers and product designers down to the Met Office headquarters in Exeter for two days full of meetings with experts in this field.
Besides providing operational and industry-specific weather analyses, the Met Office is an active research institute employing scientists working across the spectrum from daily weather to long-term climate trends. While using ECMWF forecasts as input for their predictions, they also build their own independent models.
We learnt a great deal in this two-day visit and couldn’t possibly summarise everything in this blog post but would like to highlight some key topics. First we look at how forecasts of different time scopes are modelled followed by an explanation of how ensemble forecasts can help quantify uncertainty. Next we turn to the difficulties in modeling soil moisture and finally we share our learnings on modeling wildfires.
Care should be taken not to conflate weather and climate: whilst weather refers to specific short-term effects, climate refers to more general long-term effects. Cervest works at the medium- to long-term end of this spectrum, so we have a great interest in better understanding how both these forecasts are produced.
The approaches to modeling and interpreting results in these two cases are quite different. Shorter weather forecasts can afford to neglect slow-moving climate effects (e.g. carbon cycles, sea ice changes, emissions pathways), which are crucial to climate models running over long time horizons. Furthermore, weather forecasts may be run with shorter timesteps and at a higher resolution than climate forecasts, while being less sensitive to errors in the assumed starting state of the model. This means that whilst weather forecasts can make quite specific predictions, climate models are generally limited to broad predictions.
Sub-seasonal forecasts lie between weather and climate forecasts, attempting to predict weather with an accuracy and specificity similar to that of weather forecasts, but over longer time horizons of up to around 6 weeks. These are of particular relevance to Cervest as they allow us to flag up weather risks well ahead of time, enabling decisions that minimise their impact. These forecasts are generally trickier to get right than more short-term weather forecasts or climate forecasts: whilst a lot of the signal in short-timescale weather effects is lost when predicting more than a week or two out, this period is also not sufficiently long for long-timescale climate effects to provide much signal. Nevertheless, sub-seasonal forecast quality has improved in the last few years, especially in winter months. This is largely due to improvements in modeling the North Atlantic Oscillation, a fluctuation in atmospheric pressure that determines whether we see a wet and warm winter or a dry and cold winter. Recent machine learning approaches have also shown promise to enhance similar long-range oscillation forecasts or sub-seasonal forecasts directly.
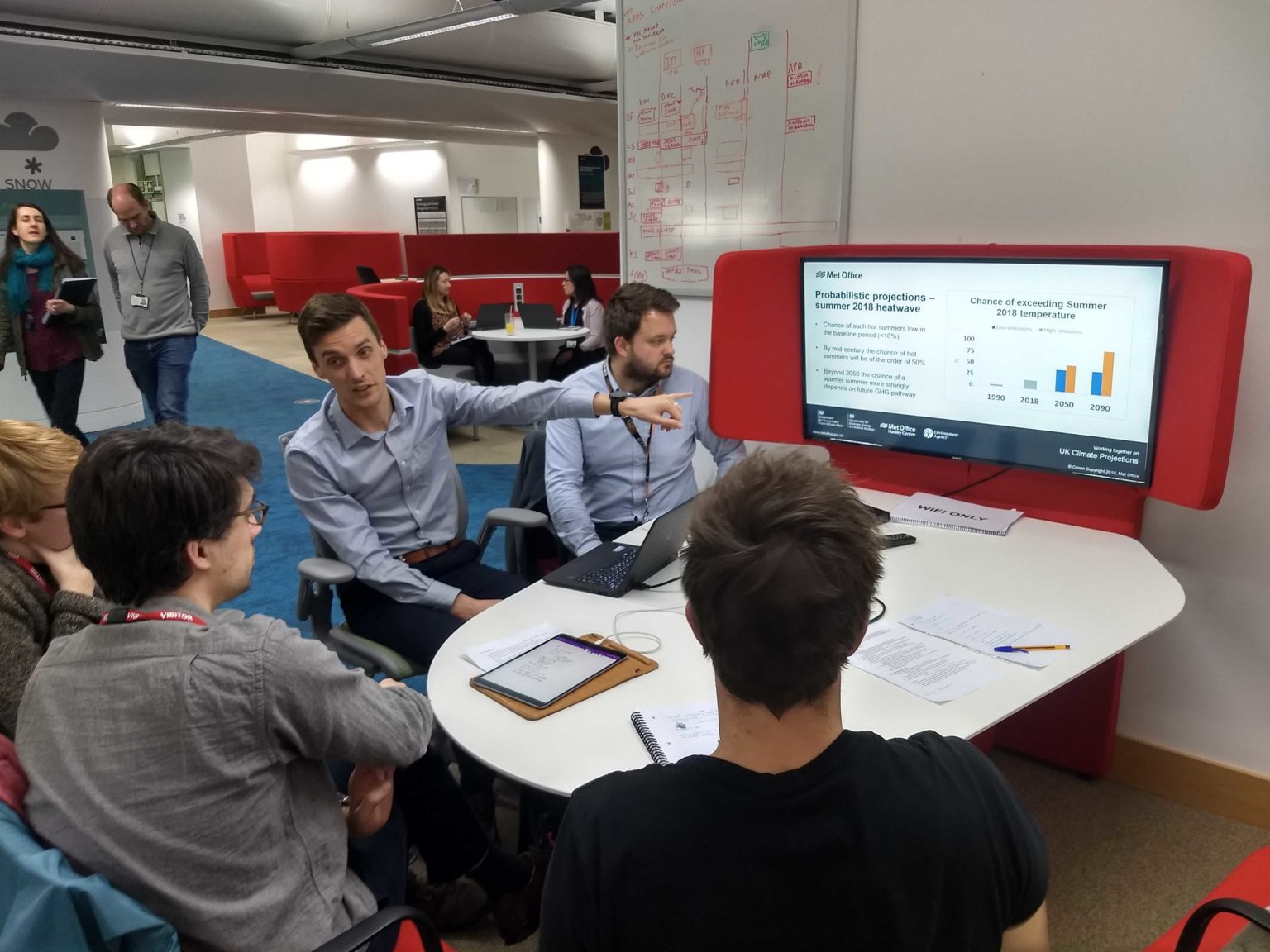
Joe Osborne, a climate expert at the Met office, demonstrating worrying future trends in heatwave occurrence
A core pillar of our approach to statistical modeling at Cervest is the proper quantification of uncertainty in our models’ outputs. Ensemble forecasts help quantify uncertainty in our inputs, which we can then propagate through our models. Since weather models are highly complex and nonlinear, extracting a simple formula for the uncertainty of model outputs is intractable. Instead, a simulation-based approach is taken, where rather than running a single model for a deterministic forecast, an ensemble of model runs is generated by running the same model multiple times with slight changes in the initial inputs and model specification itself, called perturbations. This generates a set of forecasts (one for each model run), which may be interpreted as a distribution of forecast values.
When running a model ensemble, it is important that the slight changes in each run are properly calibrated, so that the resulting distribution does not over- or under-estimate the uncertainty on the predictions. Uncertainty in the inputs and model specification is often reflected by adding some fine-tuned random noise, while more structured approaches include kinetic energy back-scatter schemes. Adding ensemble members generally gives a better representation of uncertainty but is computationally expensive. The ECMWF found that forecast quality levels off beyond an ensemble size of 50 members, which is reflected in its operational ensemble. Its forecast ensemble is therefore well-optimised to represent the uncertainty in its predictions.

Illustration of an ensemble of forecasts indicating the likelihood of different scenarios occurring. Source: ECMWF
Soil moisture is a critical component in many crop yield forecasting models so we also sat down with Malcolm Lee, developer of the Met Office soil moisture and evaporation service. He illustrated many different soil moisture measurement tools, from lysimeters to potentiometers and whilst they each have their advantages and disadvantages regarding calibration, movability and costs, all suffer from the fundamental problem that soil is highly heterogeneous. Soil composition measurements at a particular location may not generalise well to slightly different locations.
This issue of heterogeneity is magnified in global soil moisture models due to lack of knowledge of how a specific piece of land is used (e.g. is it a car park or a field?), which significantly impacts the dynamics of soil moisture. Soil moisture models may therefore produce forecasts for lots of different land-use cases, allowing users to pick the one most relevant to them, something which Cervest’s land-use modeling could help inform.
Additionally, soil moisture depends strongly on precipitation, which is highly localised and difficult to model correctly at a high resolution. Due to these difficulties in modeling soil moisture accurately, outputs should not be considered very precise, but are nonetheless useful in identifying trends and large-scale phenomena.
Wildfires are one of the natural hazards Cervest aims to model in the near future. We had the pleasure to talk with Karl Kitchen, who introduced the UK’s first wildfire behaviour prediction system.
It is important to note that this wildfire behaviour prediction system models the behaviour, not the risk of occurrence, of a wildfire. Risk of occurrence is a separate problem, highly dependent on human factors. To evaluate fire behaviour, different stages are identified: initial ignition, spread, ignition of floor-level organic material, and ignition of plants and other organic material. Humidity is a significant driver of both initial ignition and the ignition of plants and organic material, whilst the spread of fires is driven primarily by wind speed and direction.
Based on these stages, a set of indices is constructed allowing experts to predict how a wildfire might develop. However, the usefulness of any particular indicator can vary by both geography and season. Alternatively, machine learning approaches have been proposed to infer wildfire risk
The people mentioned in this article are only a few of the many experts we had the opportunity to talk with during our trip. We covered many more scientific topics and also learned a lot about technical infrastructure and visualisation. We left with a much clearer understanding of the data we work with on a daily basis and a greater appreciation of the challenges and complexity of weather and climate forecasting. All the people we met at the Met Office were extremely kind and helpful, with a special shoutout to Steven Davey and Alasdair Skea for guiding us through the days.
About us: We are Owen and Lukas, part of the Cervest Science team, a group of statisticians, machine learning & natural scientists, and software engineers. We aim to solve urgent problems within Earth Science through application of machine learning tools and techniques.
If you’re interested in what we do, then please head over to our careers page!
Share this article
Our latest news and insights
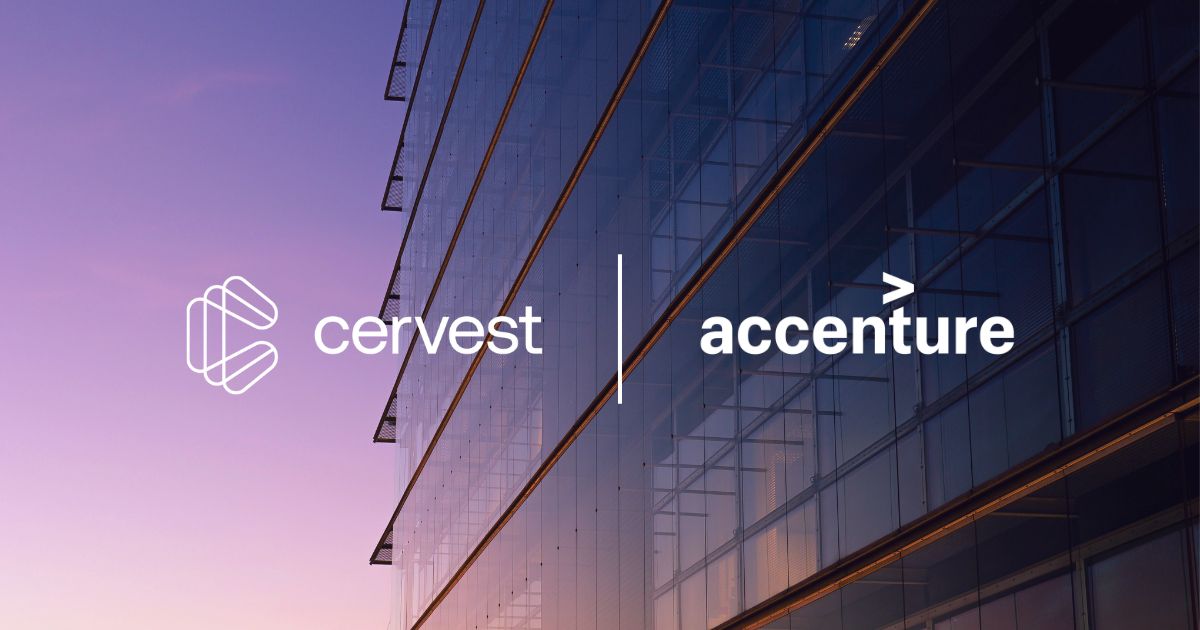
Accenture and Cervest collaborate to bring innovative solutions to clients seeking resilience amid increased climate risk
Read more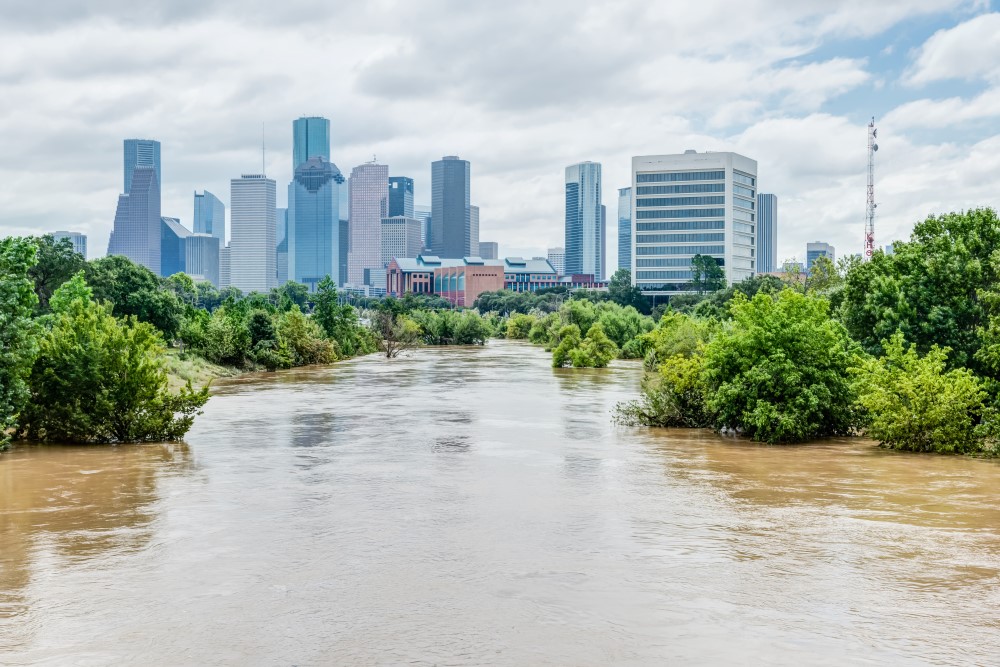
What is climate intelligence and why do businesses and governments need it?
Read more